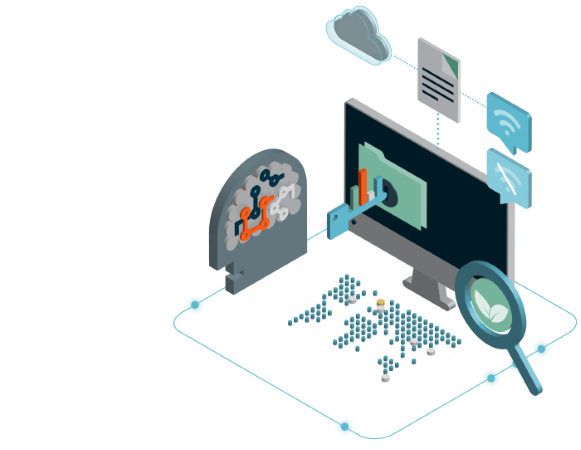
A foreword from Mark Taylor
The potential impact of AI in all sectors of the economy has come sharply into focus with the ready availability of powerful generative AI tools. It has put a spotlight on the ability of this transformative technology to boost efficiency and productivity. Boardrooms are exploring how to harness the opportunities, but also aware of the need to address the associated risks.
Understanding and managing the legal and compliance risk around AI can seem complex. It is a pervasive and flexible technology, with applications in every sector, and at any point in the supply chain.
In practice, we are supporting an increasing number of clients conducting AI audits to ensure that they have mapped out their use of AI and understand both the risks and potential mitigations. As policymakers increasingly focus on transparency around both the functioning and use of AI, AI audits are becoming a significant risk management tool, and can ensure that AI deployment aligns with wider ethical and governance considerations.
The breadth and depth of our international experience in advising on legal and regulatory issues around AI is hard to match. We would be delighted to discuss how these issues apply to your business and indeed to support you in your exploration of the transformative potential of AI.
- Mark Taylor, Partner, Head of Digitalisation, UK
What risks need to be considered by a business using artificial intelligence?
The rise of artificial intelligence (AI) applications in businesses across the economy is both an evolution and a revolution.
AI is not new. Machine learning has been increasingly embedded in our everyday devices and software for some time. Our phones predict the next word as we type a text. Our communications applications can write a simultaneous transcription of a video call. Our email services can highlight questions that we haven’t answered, or suggest blocking out focus time in a busy diary. Webpages in an unfamiliar language can be translated almost instantly. Personal profiles are used to show us targeted online advertisements. Online services recommend the next video or TV series to watch.
Yet at the same time, there has been an explosion of activity and interest in AI. The release of ChatGPT in November 2022 plus high-profile debates on the longer-term pros and cons of AI have pushed this technology to the top of boardrooms', governments' and regulators' agendas. The power and potential of systems that can create polished and creative written and visual content is very clear. Automation of more complex, knowledge-based areas of human activity is suddenly feasible. And these systems can go beyond mere automation of existing human tasks to perform functions which cannot be performed by humans.
In addition to monitoring emerging AI-specific regulation, there is a wide range of current legal and compliance risks that need to be taken into consideration.
What is AI?
Who controls the AI tool?
Emerging AI-specific regulation
Input risks
Output risks
ESG considerations
AI audits and impact assessments
Practical considerations
What is AI?
The current focus of interest and development concerns machine learning, a type of AI that partly writes and adjusts itself. This is achieved through an iterative "training" process, passing often huge quantities of data through the system. There are various types of machine learning system.
Some of the most powerful generate vast, complex and detailed maps of the patterns in the data within a structure known as a neural network. Each new piece of data passed through the system refines it further, adjusting individual settings within the network to make the model progressively more accurate. These adjustments are made automatically by the system, based on mathematical calculations of how to optimise the settings. Modern neural networks can be huge, with millions, billions or even trillions of individual settings that are calibrated and recalibrated by reference to each piece of training data.
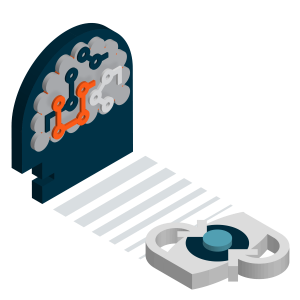
A machine learning system has no wider "knowledge" or frame of reference beyond the model created from the training data. The quality of the data therefore drives the quality of its outputs. The model itself does not retain its training data, and its outputs are not generated by searching for the right answer amongst the training data. Instead, the system generates the right answer drawing from its complex model honed by the training data – the answer is a prediction of the right response. Note, however, that training datasets may well be retained alongside a trained model. In some applications, the retained training data is searched to find sources to match and back up the answer that the model has (separately) generated.
"Foundation models", also known as "general purpose AI models", are an emerging category of machine learning systems that have been trained on hyperscaled, unlabelled, unstructured datasets that have not been curated for a specific task (but often web-scraped from publicly available internet sources). As a consequence, these models have a flexibility in application that the previous generation of AI models do not have. For example, a foundation model such as GPT-4, trained on language and text covering a vast range of topics, can be used to answer questions, to write software, to act as an interface between a non-technical user and a task needing technical prompts and instructions etc. Rather than needing to train a new AI model for each new task (as was the case previously), foundation models become a core building block for building end applications to fit many different and varied use cases.
One of the highest profile examples of applications built on an underlying foundation model is generative AI. Powerful, publicly accessible systems, which generate text or images (think ChatGPT, DALL-E, or Midjourney), are currently a particular focus as they have become so effective at creating content that is often difficult to distinguish from that created by humans. The scale of the models mean that they are often deployed "as a service" via cloud service providers, rather than being built from scratch or copied onto local servers.
From a legal risk perspective, the way in which a system has been procured, how it was trained, how it is deployed, the application that it is used for, the point in the supply chain at which it is used, and whether it is used for automation or as an augmenting tool will all play into applicable risk levels. It is worth noting that generative AI can throw up additional layers of risk that we will highlight.
Who controls the AI tool and scope for contractual protection?
As with all software, the degree of control that a business using AI will have over how it functions ranges from complete control to almost none, depending on how it is built, trained and accessed.
Where AI is developed specifically for the business by an external developer; again, there will be extensive control, subject to the terms of the development contract.
At the other end of the scale, some AI tools are cloud-based systems available "out of the box", already trained and able to be implemented immediately. The degree of control for a business customer using the system and the scope to negotiate terms and conditions of use are likely to be minimal. Procurement due diligence may identify risks that could be mitigated, but other risks will present a "take it or leave it" choice.
Publicly available AI systems such as ChatGPT fall in this category, along with AI that has been integrated into the functionality of standard enterprise software. Terms and conditions of use should be checked for obligations flowing from the licence but are likely to offer minimal protection, warranties, guarantees or redress. Many of these systems are also available on a paid-for enterprise licence. This may create additional protections for the business user, although there is generally still only limited scope for negotiating around standard terms and conditions of the enterprise licence.
It is also important to recognise that the open access market for AI solutions is as significant in the AI field as it is in relation to open source software. The vast majority of open access AI is obtained via online repositories such as GitHub, Hugging Face or Linux Foundation and is licensed under standard open source terms. Although open source elements in an AI system will be free of charge, they are not free of obligation. It is important to understand which open source licences are in play, and their terms. Some – known as "copyleft" licences – impose an obligation that software (known as a derivative work) that has been built using the open source element must in turn be open source and freely available. Clearly, this needs to be flagged if a businesses wishes to treat a valuable AI system as proprietary, or to charge for licensing it.
Many commercial AI applications are composite systems, combining elements from a selection of sources. AI software components can be built on a bespoke basis or are available from many of the major enterprise cloud services providers, as well as on an open source software basis, or can be adapted from those sources. Similarly, training datasets are available under paid-for licences or on an open-source basis. Third-party data might be used to supplement proprietary databases.
When negotiating terms for the development or use of an AI system, it is worth understanding how it is structured, where key decisions are taken in the supply chain and where control is exercised. Such insight can inform contractual negotiations around seeking warranties and indemnities and in relation to structuring liability more generally. Our experience of contractual negotiations around AI systems is that this field is too new for standard market practice to have emerged.
Emerging AI-specific regulation
AI-specific regulation is not yet in place but is a priority for many policymakers around the world.
- The EU's AI Act
The text of the AI Act was adopted by the Parliament in mid-March, (with legal and linguistic corrections accepted in April) and by the Council of EU in mid-May. However, publication of the definitive text of the AI Act in the EU's Official Journal has been delayed and is not expected until late July, meaning that it will become law later than expected, in August 2024. There will then be a staggered timeframe for when it comes into full effect (see below).
The legislation takes a tiered, risk-based approach, focused on risks to human health and safety and to fundamental human rights. It seeks to foster trustworthy AI and draws, to some extent, on the EU product safety regulatory regime (although it has much wider application). It will also cover "general-purpose AI" (including some generative AI models) which does not fit readily into the risk-based framework because the same underlying model can be put to many different applications.
A few uses of AI are considered to be unacceptable and will be banned outright in the EU, including live remote-biometric identification (such as face recognition) in public spaces for law enforcement (subject to some exceptions) and cognitive behavioural manipulation. Heavy fines of up to 7 per cent of worldwide group turnover can be imposed for breach of these prohibitions.
Some applications are considered to be high risk and will be heavily regulated if they generate a significant risk of harm to safety or fundamental rights. The high-risk provisions will apply to specified categories of AI, and to AI systems used in products that are already subject to certain EU product safety regulations. Requirements will include data governance, extensive technical documentation and record-keeping, transparency for users, human oversight, accuracy and security. High-risk systems will need to be assessed, certified, registered and will be subject to a formal enforcement regime with enforcement at national level, including powers to impose significant fines of up to 3 per cent of worldwide group turnover.
Limited-risk AI will mainly be subject to transparency requirements to ensure that people know that they are interacting with an AI (such as a chatbot), with AI-generated content (such as deep-fakes) or that an AI system is monitoring their reactions etc. Other AI applications will be unregulated.
In addition, "general-purpose" AI will be regulated, being models that are trained on a large amount of data, are able to perform a wide range of distinct tasks and can be integrated into a variety of downstream AI systems. All general-purpose AI will be subject to certain transparency requirements to make sure downstream providers can in turn comply with their own AI Act obligations. General-purpose AI considered to pose systemic risk – essentially the largest scale models at the leading edge of AI research – will face a further tier of regulation, focused on safety, cybersecurity and environmental considerations.
"Compliance by design" will be important, because retrofitting compliance into technology can be expensive and disruptive. Now that the text of the legislation is settled, businesses will need to understand which of the AI systems that they have developed, supply or use will be subject to the AI Act; what new compliance obligations will bite on the business and its supply chain; and what changes will be needed to bring the business into compliance.
The prohibitions on specified categories of banned AI will come into force after six months – early 2025. The provisions for general-purpose AI will come into force after 12 months – late summer 2025. The bulk of the legislation, including the provisions on specified categories of high-risk AI and on transparency for low-risk AI, will come into force after 2 years – late summer 2026. Where AI is categorised as high risk because it is already subject to certain EU product safety regulations, the AI Act will come into force after three years – late summer 2027.
The AI Act will apply alongside existing areas of regulation that already apply to AI. A number of data protection regulators, for example, have already been engaging with various public generative AI tools. Relevant AI tools will fall within the jurisdictional scope of many areas of new or updated EU digital regulation.
- The EU's AI Liability Directive
The status of this legislation is uncertain at present, pending announcement of the legislative programme of the new Commission, to be appointed by the newly elected European Parliament.
To reinforce the regulatory regime under the AI Act, the EU proposed to facilitate private actions to secure redress through the courts for harm caused by AI.
The AI Liability Directive would simplify the legal process for proving that someone's fault in relation to an AI system has led to damage. The new rules would retain the usual fault-based approach to non-contractual liability across EU Member States, but make two changes:
- in circumstances where a relevant fault has been established and certain requirements are met, a "presumption of causality by AI" would be triggered. The presumption would be rebuttable but would operate to shift the burden of proof onto the defendant to show that the AI system did not cause the harm in question; and
- for AI applications falling within the "high risk" category to be introduced by the AI Act, claimants would be entitled to ask a court to order disclosure of "relevant evidence", which could include compliance documentation required under the AI Act.
These changes would then need to be implemented at national level across the EU Member States but would be likely to increase the risk of damages litigation.
- The UK's AI Governance white paper
The UK is taking a markedly different approach to the EU's AI-specific legislation. The AI white paper of March 2023 proposed five high-level principles that will be informally issued by the UK government in order to guide the application of existing regulation by the existing regulators, exercising their current powers within their existing jurisdiction. This approach was confirmed in the government's consultation response in February 2024.
The five principles cover the need for:
- Safety, security and robustness.
- Appropriate transparency and explainability.
- Fairness.
- Accountability and governance.
- Contestability and redress.
The regulatory risk flowing from this approach is, therefore, simply an extension of existing risk, but in a new context. The consultation response confirmed that no new legislation or powers are planned, though the government has indicated that this may change as and when AI regulatory gaps are identified.
It is clear that a great deal of existing regulation already applies to AI applications. A number of UK regulators are already actively engaged in understanding how AI fits within their area of expertise. For example, the financial services regulators are exploring the safe and responsible use of AI in their sector, while the communications regulator, Ofcom, oversees safety of online content, often powered by AI. Economic regulators such as the Competition and Markets Authority (CMA) and the Information Commissioner's Office (ICO) are actively engaged in understanding and issuing guidance on the interface of their areas of jurisdiction with AI. The Digital Regulation Cooperation Forum convenes these and other digital regulators and is taking on a significant role as a hub for AI expertise and skills. At the request of the government, various regulators have published their strategic approach to regulating AI (available here), including the ICO, the CMA, the Health and Safety Executive, the Office for Product Safety and Standards, Ofcom, the Financial Conduct Authority, the Medicines and Healthcare products Regulatory Agency and Ofgem. Businesses in a regulated sector should monitor this aspect of the regulator's activity. The reports are expected to feed into a better understanding of the regulatory coverage of AI-related risks in the UK.
On the one hand, the UK approach will be much less onerous than the EU's AI Act regime and may suit start-ups and small and medium-sized enterprises much better. On the other hand, businesses wishing to sell their AI offerings into EU markets will need to comply with the AI Act in any case.
AI regulation and support in the UK may change after the UK election in July 2024, although we do not expect a comprehensive regulatory framework similar to the EU's AI Act.
- International AI policy
The surge in interest in AI has created a sense of urgency among governments and policymakers in many countries around international AI regulation. The compliance period under the EU's AI Act means that it will be a couple of years before the EU's AI Act will be fully enforceable, so the European Commission has created the "AI Pact". This a voluntary code of conduct with major global tech businesses, who are encouraged to adhere to the AI Act requirements on a voluntary basis before it is formally in force, and to share emerging best practice around AI Act compliance.
The UK is similarly active in multinational discussions. The Atlantic Declaration between the UK and US includes a commitment to working together on targeted international action to ensure safety and security around AI. The UK hosted the first global summit on AI safety for "frontier models" in November 2023, with a second taking place in South Korea in May 2024. The UK, US and EU are all involved in the G7 Hiroshima AI process, which has published a set of principles to ensure safer generative AI and a voluntary code of conduct for AI developers.
It is not yet clear how, or whether, these multinational initiatives will translate into compliance requirements for businesses.
Input risks
Notwithstanding the absence of AI-specific regulation yet, AI is not currently unregulated and there are a variety of current compliance risks. As regards risks flowing from inputs into the AI system, there are two broad areas to consider: the training data and the user inputs.
- Training data – bias and discrimination risk
AI systems are only as good as their training data – "garbage in, garbage out". Understanding the profile of its training data is therefore a key aspect of due diligence on the suitability of an AI tool. Ideally, training data should be appropriately aligned to the intended use of the tool. The EU's AI Act includes an obligation that training data should be relevant, sufficiently representative, and to the best extent possible, free of errors and complete in view of the intended purpose. It must have "appropriate statistical properties" for the people that the AI tool will be used for. The dataset must also take into account the "specific geographical, contextual, behavioural or functional setting" within which the AI tool will be used.
Specific legal risks that can flow from training data that has been poorly curated include bias and discrimination. An AI system has no wider knowledge than its training data. If the training data is skewed towards (or against) a particular social, racial or cultural profile, for example, the outputs that it generates may be similarly skewed. Some forms of bias can amount to illegal discrimination under equalities legislation. Bias may result in unfairness that could infringe consumer protection law where the AI system feeds into consumer-facing products or services. Even where the bias is not illegal, it can generate material reputational risk.
- Training data: data protection risk
If training data includes information about identifiable individuals, it is likely to fall within the scope of the EU and UK versions of the General Data Protection Regulations (GDPR). This is a risk for all AI where information about people might form part of the training dataset.
Data protection risk is particularly acute in relation to generative AI. Given the huge amounts of web-based data understood to have been used to train some of the well-known generative AI systems, it is inevitable that this training data will have included personal data and potential data privacy risks. While web-scraped data including information about or images of real people might be lawfully used to train AI in some jurisdictions, the practice faces challenges in the EU or UK, where data protection rules tend to be stronger than elsewhere.
At the most basic level, personal data cannot be processed without an appropriate lawful basis. The ICO took action against a UK hospital trust for failings, including to inform and obtain consent from patients to use their medical records in the development of a commercial AI system. European data regulators (including the ICO) have published a joint statement highlighting the "significant privacy concerns" arising from automated web-scraping. The AI Act will prohibit AI systems that build training data for facial recognition systems using untargeted scraping of facial images from the internet or CCTV footage.
The EU and UK GDPR include requirements around automated decision-making which apply where AI is deployed to take decisions that have a legal or similarly significant effect on individuals. While automated decision-making in many areas will not typically fall within the scope of these provisions (for example, automated personalised advertising placement), they may apply where AI is taking decisions with more material ramifications such as recruitment, loan applications, etc. In such cases, it will be necessary to have a lawful basis for using the AI tool; transparency about use of the tool; simple mechanisms for the individual to request human involvement or to challenge the decision; and regular ongoing checks that the system is working as intended.
Compliance risk in this area is, of course, much wider and extends to requirements to undertake a data processing impact assessment (DPIA), that it must be possible to withdraw consent, to have errors corrected, or to have data deleted, and to comply with overarching principles including transparency, accuracy and fairness for those whose data is processed. Requirements around the international transfer of data must also be complied with.
The UK had planned to reform the UK GDPR and the Data Protection Act 2018 with the Data Protection and Digital Information Bill. This would have included reforms to provisions on automated decision-making to provide that safeguards must be in place around the use of this technology, rather than stating that it is prohibited unless it falls within one of the exceptions. The bill would also have made reforms to international transfers of personal data. However, it was not passed before Parliament was dissolved in May 2024 for the general election. It is not clear whether these proposals will be revived by the next government.
The ICO has issued extensive guidance around the use of personal data in AI tools including a series of consultations that will feed into updating its guidance. A number of EU data protection authorities have been active in querying GDPR compliance of high-profile publicly available AI systems. For example, use of ChatGPT was temporarily suspended in Italy not long after its launch while queries were addressed by the Italian regulator around GDPR compliance. The Austrian data protection authority is currently reviewing a complaint that "hallucinations" mean that ChatGPT does not comply with the GDPR's accuracy principle or with an individual's rights to rectification and deletion of personal data where that data is inaccurate.
- Training data: IP risk
A second area of legal risk around training data is the possibility that it is subject to third-party intellectual property rights. Again, this is a risk for any AI system but is a particular concern in relation to generative AI, or any form of AI trained on web-scraped data. A dataset gathered by web crawler software 'bots' will carry copyright and database right infringement risk if it includes any content obtained without appropriate permission from the content owner. This is an area where private litigation is on the rise, with both individuals and businesses taking action to protect their intellectual property from unauthorised use.
In the UK, there are some legal exceptions to both copyright and database right protection, for example, the text and data mining exception to copyright. However, these are generally considered not to extend to copying data for commercial purposes, so are difficult to apply to web-scraped training data used for commercial AI. New legislation may be issued to find a middle path between AI developers' need for training data and the rights of those with IP rights over that data. However, any such change is unlikely to happen quickly.
In the EU, there is an exception to the copyright rules for text and data mining conducted for any purpose, unless the rightsholder has expressly opted out of this exception. Many terms of use for websites include such a reservation. Where possible, confirmation should be sought that all necessary licences to use the training data have been obtained.
The provisions in the EU's AI Act that will regulate "general purpose AI models" (the term used in the Act for foundation models) include an obligation on model providers to put in place a policy to ensure the respect of EU copyright law, including where a website provider has opted out of the EU copyright exception for text and data mining.
- Training data: algorithmic disgorgement
There have been a small number of cases in the US where infringements in gathering or using training data have led to regulatory sanctions requiring not only the training data to be deleted but also the trained algorithm – a remedy known as "algorithmic disgorgement". This onerous sanction flows from how machine learning is built. These systems do not retain their training data but model the patterns across the training dataset. Deleting the training data, therefore, would not remove the benefit of having obtained or used data illegally in the system.
The US Fair Trade Commission (FTC) has, therefore, ruled in a small number of cases that the trained algorithm itself should be deleted, including its settlements with WW International in February 2022, Everalbum in May 2021, and the order against Cambridge Analytica in November 2019.
We are not aware that this remedy has been imposed in Europe, but it cannot be ruled out where it would be within the scope of regulators' or courts' powers to remedy harm.
- User inputs: confidentiality and wider use
Where an AI system operates on the basis of some form of user input, it is important to understand where that information goes to and how it is used.
Particular care is needed around confidential information where the AI system is a public one, accessed from the cloud. This concern certainly applies to many generative AI systems. It is known, for example, that questions and data put into the public version of ChatGPT by users are logged and retained by OpenAI and are used to adapt or refine the system. Confidential information, business secrets, trade secrets, etc. may need to be withheld from these systems or redacted before being entered, in case confidentiality is compromised. Businesses subject to obligations around client confidentiality should carefully consider whether to put client information into such systems. It may also be a breach of business' contracts with clients/customers.
It is also important to understand whether the system provider intends to use input data for any wider uses. For example, will it be added to training data for the same system or for other uses?
Output risks
Output risks around AI systems may be based on black-letter law or looser risks flowing from ethical considerations
- IP risks
Not all AI systems generate outputs that might qualify for protection under IP rights: it is, of course, possible to create value, speed and efficiency without doing something new, creative or innovative. Where a system is generating text, computer code or visual content, however, securing copyright over outputs may be important. Where it is generating innovative proposals around technical products or processes, patents may be in play.
In the UK and EU, where content is created by a human with assistance from an AI tool, the human will own any copyright, as long as the work expresses original human creativity. However, the position may differ if an AI tool has been set up to churn out content.
In the UK, there may still be copyright protection available for automated content. Copyright would belong to the person who undertook the arrangements necessary for the work to be created. How these provisions apply to generative AI systems has not yet been tested in the courts. In the EU, it is generally considered that there is no copyright for AI-generated creative works. The best way to ensure copyright protection for AI output is therefore to ensure that the system is used as a tool, not as an automated flow of content.
As regards the patentability of AI-generated innovations, both the EU and UK courts have ruled that an AI system cannot be an inventor for the purposes of filing a patent. By contrast, inventions by humans that use AI as a tool will be patentable.
In the UK, the Supreme Court has confirmed that an invention generated by an AI system without any human contribution cannot be patented by the owner of the system if the system is stated to be the inventor. The position is currently similar in the EU, where the European Patent Office has ruled on the matter.
In practice, therefore, where creative content is being generated by an AI tool, the risk that IP rights might not be secured is mainly in play where the AI is being used not merely as a tool in the human creative or inventive process, but to replace human authors or inventors or reduce their role so that they play no significant part.
As a separate point, IP input risks can flow through into output risks. The risk that AI outputs could infringe the IP rights of a third party should be mitigated by proper curation of the training dataset. If all training data has been appropriately licensed, then the risks of infringing outputs should be much reduced (although terms of the licence should be checked to verify whether it deals with ownership of IP rights in the output from the trained model). Again, particular caution is needed in relation to generative AI and any other AI system that has been trained on web-scraped training data. Where the provider of the tool or of the training dataset has given warranties that all appropriate licences have been obtained in relation to the training data, it is sometimes possible to secure, in addition, an indemnity against liability arising from outputs that infringe third-party rights.
- Accuracy, hallucinations and bias
The quality of an AI system's outputs is driven by the quality of the training data that the model has been built on – garbage in, garbage out. As well as checking the quality of curation and choice of the training data, it is important to check the outputs from the AI.
The training process will typically involve refining the system to an acceptable level of accuracy in its answers. However, the nature of neural networks means that unexpected answers can still crop up. This might be because the patterning in the model has spotted something that humans hadn't previously considered. Or it may generate an "edge case" – a reasonable answer but at the margins of the possible outputs. Monitoring outputs on an ongoing basis will be important to ensure that the risk around accuracy is understood and mitigated.
"Hallucinations" from generative AI tools have been much discussed. Any AI system can generate an incorrect response, but there is an enhanced danger of overlooking inaccuracy or fiction presented as fact when an answer is very eloquently phrased and entirely convincing-sounding – which is the particular skill of systems such as ChatGPT. The simplest mitigation of this risk is for someone sufficiently knowledgeable on the topic to check all output, for applications where accuracy is important.
In addition, it is important to understand whether an AI system remains dynamic – self-adjusting and changing – after it is put into operation. Some machine learning systems are dynamic while they are being trained, but typically the self-calibration process ceases once they have been deployed. Other systems continue to learn from data passed through them (for example, from new data passed through them or from feedback on outputs). Such systems will need more active ongoing monitoring to understand how they are changing. Generative AI systems tend not to be dynamic – the underlying foundation (or general purpose) model is static – but there are further ways in which their output can be adjusted in the "back end", such as through a process known as Retrieval Augmented Generation or "grounding" which aims to contextualise model output by reference to data provided subsequently by users. Some of these changes can be controlled by the user but others may be undertaken by the system provider. This needs to be monitored, to understand how such changes could impact on functionality and outputs.
The specific legal risk around accuracy may flow from various sources. Where the output from the AI tool impacts on the business' customers, there may be contractual provisions that create (or exclude) obligations around accuracy or quality of products or services provided. Where accuracy of outputs could impact on product safety or quality, product regulation may be in play. Inaccuracy and bias in outputs can infringe consumer protection law in consumer-facing products or services, particularly in relation to chatbots.
Where the AI has been trained on personal data, obligations to remove or correct inaccurate outputs about individuals may apply and the wider requirements of the UK and EU GDPR will apply, even if accurate, to any such content. Inaccurate outputs about identifiable individuals could, moreover, create defamation risk. Under the AI Act, high-risk AI will be required to operate to an appropriate level of accuracy.
- Transparency, explainability and the 'black box'
The neural networks that underpin an AI system can be immensely complex and are typically opaque. Explaining the outputs of the model in terms that correspond to how a human would think about the same problem can sometimes prove to be an intractable task. First, the model is based on maths (statistics-based predictions of the least wrong answer) not human-style reasoning (weighing relevant facts, considerations, experience, etc. to reach a conclusion). But the difficulty also results from the potentially massive complexity of the neural network. The potential difficulties in understanding and explaining these systems is known as the "black box" problem.
The general approach of policymakers and regulators is to ensure that a lack of explainability in some black box systems should not operate to enable a denial of responsibility – a defence to a liability claim or regulator's query on the basis that "the AI did it" is unlikely to succeed. The UK white paper's approach to transparency is that regulators should be able to obtain sufficient information about an AI system to perform their functions. However, given the technical difficulties around AI explainability, it notes that transparency and explainability are not absolute requirements but should be applied proportionately to the risks in play. The ICO has issued guidance developed with the Alan Turing Institute about explaining AI that processes personal data.
As with other output risks, the first consideration around explainability is whether the AI system impacts on third parties. Again, there may be contractual provisions creating (or excluding) obligations dealing with the transparency and explainability of outputs. The AI Act will create overarching transparency requirements for high-risk AI, although again the obligation will be tempered to a type and degree of transparency that is appropriate for the system in question. Transparency is also required under the EU and UK GDPR, including in relation to automated decision-making.
In addition to transparency in the sense of explainability, transparency can also refer to letting stakeholders know that an AI system or outputs are in use. The AI Act will impose transparency obligations of this type on certain lower risk systems. This will include informing natural persons when they are interacting with an AI system such as a chatbot unless obvious from the context. Emotion recognition systems, biometric categorisation systems and systems generating synthetic content or deep fakes are also areas where transparency about use of the AI system is required.
Overall, the regulatory trend is towards making sure that people know when they are engaging with AI outputs or content. This is driven by the desire to ensure that AI technology is trusted and therefore widely adopted.
- Cybersecurity and robustness
As with all IT systems and software, cybersecurity around AI systems is important. This may be an operational risk to be managed by the IT team, rather than a legal risk, although legal obligations to ensure cybersecurity can flow from critical infrastructure legislation and from data protection legislation.
Where an AI system is being accessed via a connection into third-party servers (including cloud-based providers), the usual considerations will apply to ensure that that connection does not create vulnerability as an additional entrance point into the business's systems. This is again partly an operational issue but can be reinforced with contractual provisions and benchmarks for performance.
Additionally, the ready availability of generative AI systems able to generate convincing text in a wide variety of languages means that hackers can easily create sophisticated communications, such as phishing emails designed to trick IT system users into allowing unauthorised access.
ESG considerations
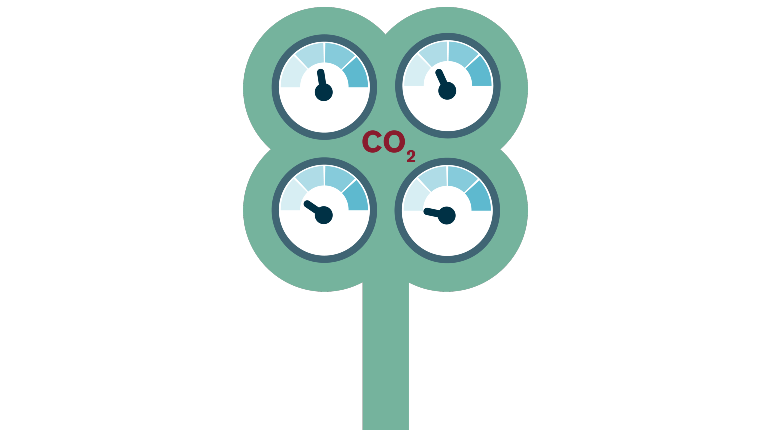
Environmental risk
The emergence of powerful AI systems is the result, in part, of the increasing scale and reducing cost of computer processing capacity. The latest generation of machine learning systems are very large – they tend to run on hyperscaled data using complex models. Under the AI Act's general purpose AI provisions, energy consumption may be one of the criteria in assessing whether an AI model poses systemic risk. A voluntary code of conduct is planned to ensure energy efficient programming, design, training and use.
The environmental impact of the energy required to power such a scale of processing cannot be overlooked. The European Parliament has estimated that the share of the world's electricity consumed by data centres, digital networks and other information technology was 7% in 2021, projected to increase to 13% by 2030.
Businesses using AI must consider this aspect of their environmental footprint and should investigate it as part of their procurement due diligence. That said, the major cloud providers have all invested heavily in renewable energy for their data centres and wider operations and in their net-zero strategies more generally. An AI system should not therefore necessarily have poor environmental performance, but this risk should be checked.
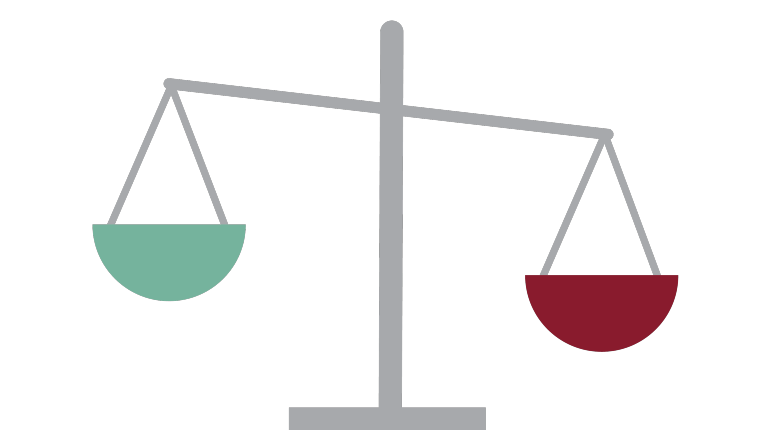
Social risk
A key aspect of the social risk of AI is its impact on a business's workforce.
Introducing automation through deployment of AI may in turn necessitate reskilling, upskilling or restructuring of the workforce. Where AI replaces human labour altogether, reskilling may be needed to redeploy staff into new functions within the business. Redundancies may be needed where alternative roles are not available. Workforce restructuring is, of course, subject to legal requirements around process and consultation, with corresponding risk of employee disputes if those obligations are not met.
Where AI is used as a productivity tool, reskilling may be needed in how to use the tool. This may be a developing process – skills in the optimal use of new generative-AI tools for creating written content etc. are being explored across the economy. It is important to consider all parts of the workforce when designing reskilling and retraining programmes. In particular, discrimination risk could arise if such programmes are not made available and accessible to all ages of worker.
In addition to social risk, AI creates wider employment/HR risks. Where AI tools are introduced, or publicly accessible and available to staff, businesses will need to expand policies (or introduce new policies) around acceptable use of technology and internet resources to include these options, in order to ensure that responsible employer duties are being met. Some of the risks will need to be worked into policies to give staff clear guidance, including ensuring that there is no input of confidential or client information into public AI tools, or that such systems are not being used to generate harmful content. Where policies are not followed, risks of disciplinary proceedings will follow.
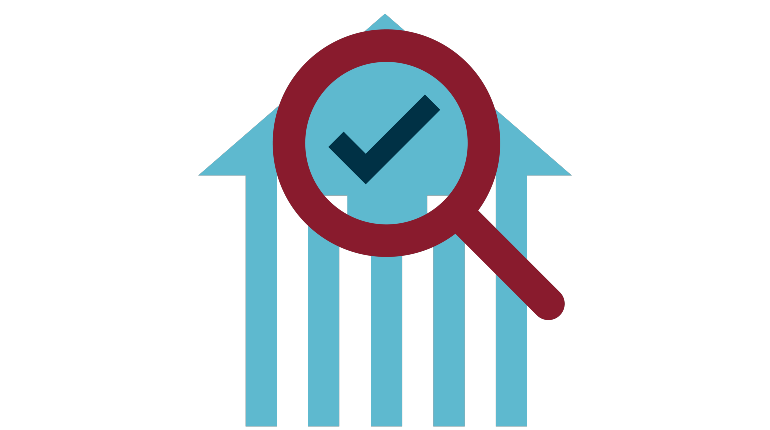
Governance risk
AI has long been a topic for ethical debate. Hollywood's portrayal of AI may be far removed from the reality of the technology as it exists today, but it has created a perception that the risks are potentially existential. Killer robots remain the stuff of science fiction for the time being, but businesses need to be aware that many of the AI systems currently available are still relatively crude, the equivalent of early working drafts. Their capabilities are expected to increased rapidly, possibly over a fairly near-term timeframe. It is therefore important to assess AI in terms of short to mid-term future developments, not simply planning for what it can do now. Policymakers and legislators have gathered at international level to discuss AI safety and have emphasised that AI needs to be safe and be trustworthy if the potential gains for society and the economy are to be realised.
Many businesses involved in the development of AI have recognised the importance of taking an ethical approach, establishing oversight bodies, policies and audit processes for their work around AI, regardless of regulatory obligations. These policies are directly relevant to their customers, driving trust in their products and services. This is not only a matter for the business itself; ensuring that the governance approach of key suppliers in a business's digital supply chain is acceptable and sufficiently aligned with its own approach is an issue for procurement due diligence.
As the use of AI across all sectors expands, businesses that do not develop AI but integrate it into their business processes or customer-facing products and services need to consider their own policy for how they wish to use AI. This will be shaped by the nature of the business concerned, its customer base, whether the AI is being used internally or in a manner that will impact on products, services or platforms provided to customers or third parties, and the business's overarching approach to customer trust, its reputation, and ethical considerations more generally.
The UK AI white paper's proposal of high-level principles to guide regulators could also be used as a steer for corporate AI governance. Many organisations have issued frameworks for ethical AI: one of the better known examples of which is the International Technology Law Association-developed Responsible AI: Global Policy Framework, which was updated in 2021, following the publication of ITechLaw's first edition in 2019. (John Buyers, partner of Osborne Clarke, is co-editor and a co-author of this work.)
AI audits and impact assessments
Many businesses are implementing a policy of conducting an audit of their use of AI, in order to understand the specific risks for an AI tool and to identify risk mitigation options.
The market for algorithmic auditing and for AI assurance techniques more generally is in its early days. The UK Department for Science, Innovation and Technology has published a Responsible AI Toolkit as well as a portfolio of case studies of AI assurance techniques across a range of sectors and AI uses, demonstrating different approaches taken to ensure that AI is trustworthy. The UK's Digital Regulators Cooperation Forum has launched the AI and Digital Hub, a multi-regulator sandbox to help digital innovators navigate regulation.
At EU level, the AI Act will require compliance assessment for AI that falls within its high-risk category. Some businesses will be able to self-assess and certify compliance, but others will need to obtain third-party accreditation of the compliance of AI used in products subject to the EU product safety regime. An AI sandbox is being piloted that will enable innovators to understand compliance requirements in practice. In addition, a "fundamental rights impact assessment" will be required for the "first use" deployment of the specified categories of high risk AI, to be repeated where any of the factors relevant to that assessment have changed. Separately, AI systems that could fall within the "high risk" category may be able to avoid the burden of the high risk regime if they are assessed by the business concerned as not posing a significant risk of harm to safety or fundamental rights.
The UK and EU GDPR include requirements to conduct DPIAs for uses of new technologies in certain defined cases as well as more generally where the processing of personal data is likely to result in a high risk to the rights and freedoms of individuals. Many AI systems will fall within the requirement to conduct a DPIA.
Practical considerations
There is an extensive matrix of legal risk around the development, deployment and use of AI that includes both existing risk and future considerations. While we have taken a broad-brush approach in exploring this matrix, risk considerations for a specific business or a specific AI system will depend on the context and particularities of the business and the system.
In practical terms, businesses should consider the following:
- Develop an overarching governance approach to your use of AI, taking into account your business's approach to ethical issues, reputation and risk exposures.
- Understand what AI the business is using and what it does. Consider both AI that has been specifically developed or procured, and wider AI tools that staff are accessing and using for their work.
- Identify whether any of the business' AI systems will fall within the scope of the AI Act. Start to plan for compliance. Monitor the approach of UK regulators that are relevant to your business.
- For generative AI and other publicly available AI tools, consider carefully whether the business' interests would be better protected by investing in an enterprise licence rather than using the free tool. Where an enterprise licence has been purchased, be very clear whether staff can also use free versions in their work. Issue clear guidance around data that can or cannot be inputted into generative AI systems, considering confidentiality, personal data, trade secrets etc.
- Consider the impact of AI on your workforce and develop reskilling or restructuring policies as needed. Issue staff policies in relation to the use of publicly available generative AI tools such as ChatGPT, Google's Bard, and popular image-generation AI systems such as DALL-E and Midjourney.
- Consider the impact of your use of AI on your supply chains, both physical and digital. Work any necessary protections, mitigations and audits into your contractual frameworks.
- Consider the impact of your use of AI on your customers. Particularly where products and services are consumer-facing, consider whether customer terms and conditions and other customer information need to be amended to ensure transparency about the use of AI in your products and services.
- Consider the impact of your use of AI on your intellectual property, where this is a significant asset class for your business. Develop policy around the use of your intellectual property in training data for AI and in relation to securing rights over relevant AI outputs. Be clear with staff whether public generative AI tools can be used, given IP input and output risks. Consider an express provision in the terms of use of your website to exclude permission to web-scrape the contents.
- Consider whether processing of personal data by AI systems is necessary or appropriate for your business. Adapt your data protection compliance policies accordingly, including securing a lawful basis for using personal data as AI training data, where needed. Issue clear guidance to staff if personal data should be put into a particular AI system, particularly publicly available generative AI systems.
- Develop a set of priorities and benchmarks against which AI tools should be audited, and put in place initial and updated audit processes for existing and new AI systems used in the business.
If you would like to discuss any of the risks raised in this article, please do not hesitate to contact a member of our international AI team, which includes the authors of this article.