Smart cities | How AI is boosting understanding of urban dynamics
Published on 8th Oct 2020
How can artificial intelligence tools support our understanding of urban environments and drive the development of smart cities? We report on recent research projects in this field
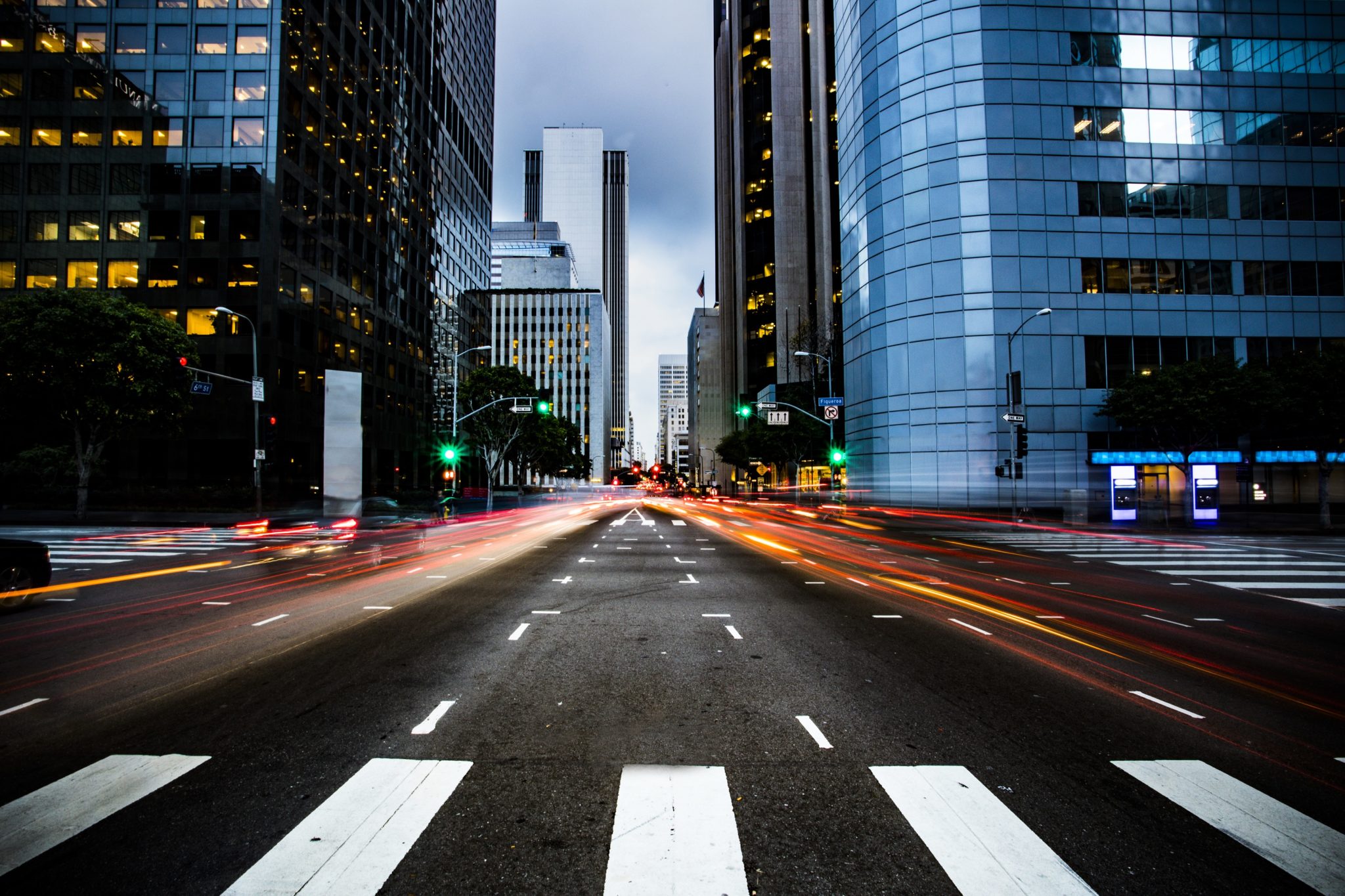
The development of smart cities is multi-layered and multidisciplinary, comparable to a huge jigsaw of interlocking, dynamic parts. Artificial intelligence is one of the key technologies which contributes to building these structures, offering insight and optimisation, while smoothing out complexity. Researchers from the UK's Alan Turing Institute (ATI) recently presented some of their work on using AI (and machine learning, in particular), to boost urban analytics. In this Insight, we report on the projects discussed in that event.
Modelling people and traffic
Mobile phone networks generate extensive data about people's movements. This is already used, for example, to report on traffic levels in map apps. However, obtaining granular data about precise movements across a city landscape requires costly measurement infrastructure. Some "probes" capture data across a wide area, but with low resolution (in the sense that data is aggregated across geographical blocks). Other probes capture much more detailed information with a tighter focus, but have more limited geographical coverage. The two sets of data can be combined, but this requires significant data storage and time-consuming analysis – and the resulting insights often come too late to be useful for managing traffic or identifying crowds.
The ATI researchers explained how they have developed an AI tool which can generate synthetic high-resolution data from low-resolution probe data. This is not the true granular, detailed information, but a prediction of it. For many purposes, as long as the prediction is sufficiently accurate, this synthetic data is a good proxy for the actual data, offering cost and time savings.
The research team's AI tool has been refined to the point that it can even handle an unexpected, very specific peak in mobile data consumption in a particular location (such as a football match on the outskirts of the city). The low-resolution data smoothed out the data spike into a much wider but flatter increase. The AI tool did not exactly predict the true spike, but did generate a data spike with broadly corresponding characteristics.
A separate project focused on modelling how people and crowds move through urban space. This can support managing crime spots or understanding where pollution hotspots overlap with areas of population density, in order to make city centres as appealing as possible. Or – as the coronavirus pandemic has brought to the fore – it can help to understand interactions for public health purposes.
Rather than treating a group of things as a whole, "agent-based modelling" simulates each element within the group separately, with small pieces of code to represent each one, with its own behaviours. This approach enables a crowd to be modelled on the basis of the individuals within it.
The challenge is to build an AI model where the predicted movements of the individuals – and therefore the crowd – are a close match for what actually happens. This is difficult to do: the alignment is often initially good, but the movements of the model typically quickly diverge from reality. In order to reduce this divergence, techniques developed for meteorology modelling are applied, feeding real-time data into the agent-based modelling to adjust it regularly and tether it closer to reality. It is hoped that this work will feed into urban digital twins, as well as facilitating short term forecasts and crisis response.
Supporting policy decisions
Many data-driven models are used by government to shape and refine urban policy.
One example models the environmental and employment consequences of infrastructure decisions. If a new road or rail link is built, or if an airport is expanded, what impact will that have at a regional level? How will the number of road journeys made change (with corresponding environmental impact)? How will the change affect job creation/employment levels (and therefore population distribution)?
A second layer to this project takes the regional analysis outputs and drills down to the local level. Forecast changes in employment levels in a particular area can be fed into local planning decisions, based on anticipated future demand for residential housing and local amenities such as supermarkets or schools.
Modelling can also ensure that the most useful data is gathered for the decision in question. For example, one of the ATI research teams discussed their work around monitoring pollution in Newcastle. Ideally, pollution-measuring sensors need to be located so that the network is not just fit for purpose but designed for purpose.
Trade-offs are inevitable because of cost constraints, but the researchers commented that what really matters for policymakers is to be able to say that no groups were ignored in reaching their decisions, whether those groups are defined by demographics or by social factors such as age, race, poverty or health. The model helps policymakers to make defensible choices.
Another ATI project supports the Greater London Authority and Transport for London. Originally centred on analysing air quality, the coronavirus pandemic shifted the focus to monitoring mobility and activity levels to understand how the "busyness" of London had altered. Data was collected from multiple sources including satellite images, ground pollution sensors, road sensors counting vehicles, traffic cameras, and CCTV systems counting vehicle types. Activity levels were assessed for the whole city, for boroughs, and at local level, as close to real time as possible.
The refocused model enabled dynamic insights into how transportation changed due to lockdown. It enables social distancing to be measured from video footage and has operated as an event detection and early warning system, helping to identify gatherings which could create contagion risk. It has also been used to optimise running, walking and cycling routes, enabling them to be designed to avoid air pollution and crime spots, and to maximise social distancing.
Osborne Clarke comment
These projects illustrate a number of the legal issues around deploying artificial intelligence. Many of these issues need to be tackled at the earliest stage so that compliance and risk mitigation is designed into the AI modelling systems as far as is feasible.
Datasets for modelling human activity should be curated with a consideration of whether identifiable information – which could engage GDPR/data privacy compliance – is actually needed, or whether anonymised data will be sufficient. Similar care is needed in the design of data collection about people's activities. Clearly, surveillance of individuals can create public trust issues as well as legal questions.
Another issue is bias, particularly where a decision-maker seeks to reach defensible conclusions taking all stakeholder interests into account. For example, using mobile phone data as a proxy for understanding human movements might exclude those who do not have a phone. In addition to the social issues presented by digital exclusion, bias can, in some circumstances, amount to illegal discrimination. As a result, gaps, errors or a lack of representation in the dataset on which an AI tool is trained can end up building legal risk into a system.
AI-generated synthetic data is a fascinating legal area. Some artificial content such as "deepfakes" can generate legal risk and even engage liability, but using synthetic data in place of real data is not an issue in itself. As well as the project to understand urban movements described above, synthetic data can be used to protect privacy. Care is needed that the synthetic data offers a sufficiently accurate proxy for the real data – how close it needs to be will depend on the situation.
Finally, the degree of interest from government in promoting AI tools for public sector use is illustrated by the "Guidelines for AI procurement" which were issued earlier this year by the UK Office for Artificial Intelligence. There is no doubt that artificial intelligence is a powerful tool in the development of urban analytics, and part of the interplay between the public and private sectors in building productive, safe, efficient, pleasant and smart cities.
If you would like to discuss any of these issues further, please don’t hesitate to contact the authors or your usual Osborne Clarke contact.