Data pooling and data integration in groups of companies
Published on 14th Jun 2022
There are various stages in the life-cycle of a dataset. First, the dataset must be created, whether this is achieved organically, or through merger, acquisition or asset transfer. Then the dataset may be enhanced through the addition of data available from external sources. Thorough analysis of the combined dataset can lead to a deeper understanding of a group's customers, their needs and interests, with resulting benefits. But understanding the limitations on data use at each of these stages is critical to the success of a data pooling project.
This is chapter 2.5 of Data-driven business models: The role of legal teams in delivering success
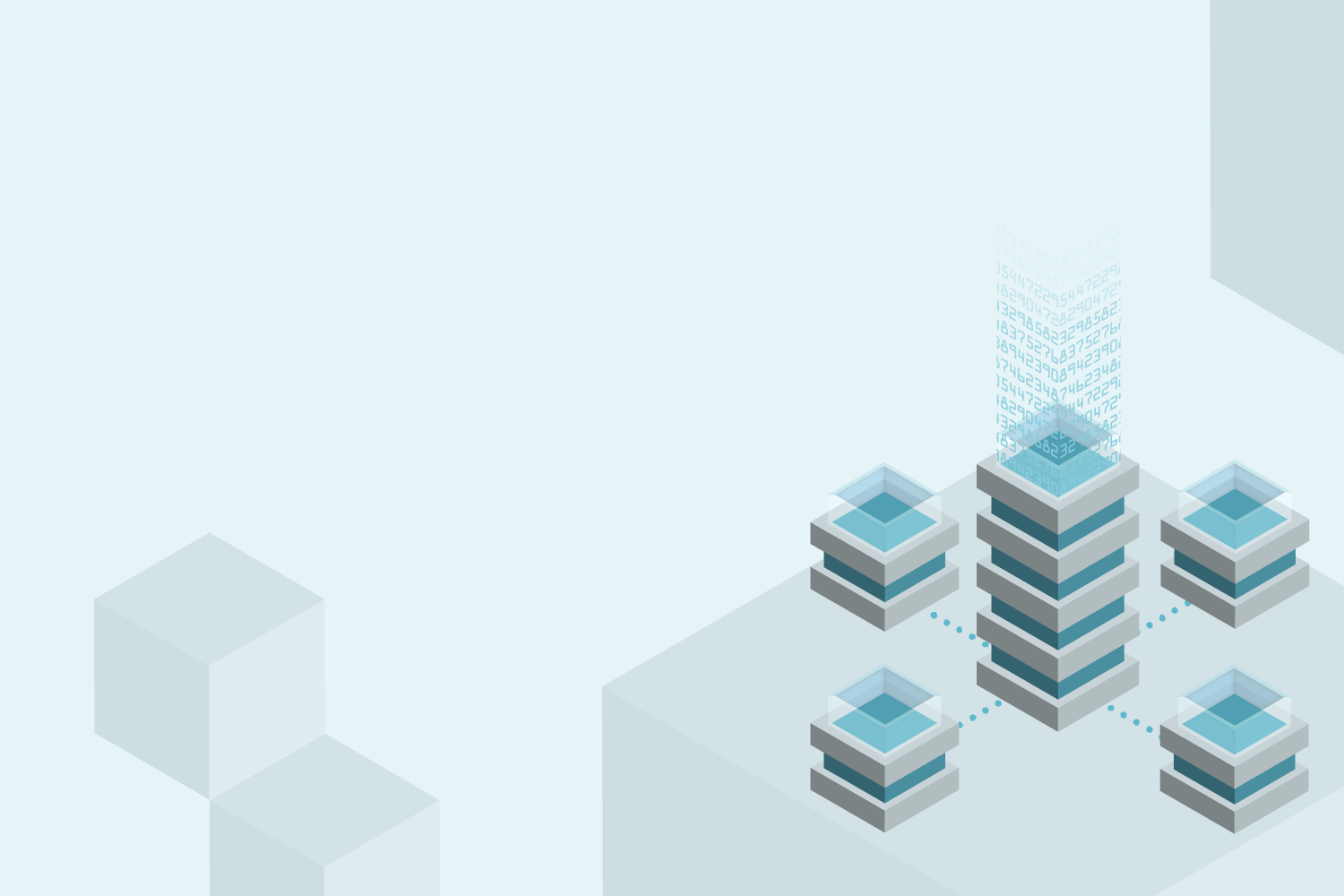
Key Takeaways
|
Building a data pool through mergers, acquisitions and other transactions
When a business wishes to build a database or expand an existing database into a data pool, it will commonly consider merging with or acquiring another company, or purchasing specific databases through asset transactions. Before doing so, it is crucial to establish that applicable laws (including those relating to data protection, intellectual property and competition) as well as contractual restrictions will not hinder or prevent a business from achieving the ultimate goal of creating a combined data pool. Conducting careful due diligence is therefore of utmost importance.
The acquisition of a database may in certain cases be subject to prior requirements and approvals. Depending on the nature of the data included in the database it may be necessary to obtain prior consent from data subjects (for example, where the transaction involves health data) or to offer data subjects the possibility of an opt-out. Additionally, where the transaction involves personal data, both the purchaser and the target company will be required to inform the data subjects of the transfer as soon as reasonably possible.
In order to avoid unnecessary barriers and delays, we often advise clients to agree joint statements and consent forms prior to completion of the transaction and to include the drafts in the transaction documentation. Another helpful strategy can be to agree arrangements for some key processes in advance, for example, about how data subjects are to be given the opportunity to supplement or correct their data prior to the transaction. Obtaining accurate and up-to-date data will contribute to an increase in the value of the database.
Enriching internal databases with data available on the market
One way in which a business's databases can be enriched is by combining the personal data held by the business internally with data from external sources, most notably statistical data. Useful statistical data may be that relating to the geographical distribution, or the socio-demographic, structural or economic characteristics of the local population. This information, once cross-referenced, can make it possible to identify new evaluation parameters that enable the business to categorise its customers into different clusters (that is, groups of customers that have similar characteristics).
Following this enrichment process, the business's clusters would not be configured exclusively on the basis of the potential client's behavioural analysis but would be redistributed by means of socio-demographic categories provided by the external source of data. This allows the business to identify new, and increasingly defined, sets of potential users to which to address more targeted and therefore more effective promotional campaigns.
This process of association is based on probability and aims to maximise the likelihood of attributing particular characteristics to a certain category of users. Such processing requires the evaluation of the company's legitimate interests and a thorough scrutiny of the new information that is selected, to ensure it is relevant to the purposes for which it is collected.
Data enriched in this way should also be subject to a limited retention period, given its probabilistic nature, subject to possible subsequent irreversible anonymisation. Furthermore, users must be adequately informed of this processing and given a right to object. From a technical point of view, systems should ensure logical and/or physical separation of information, segregation of duties, and access on a need-to-know basis only. To ensure legal and regulatory compliance of a project such as this, co-operation between the marketing, commercial, IT and legal functions is essential.
![]() Data-driven business modelsThe role of legal teams in delivering success |
Creating profiles from data pools for personalised service and marketing
The combination of databases and datasets across separate entities in a group of companies or across different business segments can very often lead to greatly improved insights into how the group's products and services are used. These insights, gained through a deep analysis of the data pool, can provide various benefits to a company: they can allow a business to improve its products and services (for example, by designing them to better meet their customers' needs), or they can be used to make advertising more targeted (for example, by better focusing on what the customer might be interested in).
However, a company can usually only benefit from these advantages if it stays within the narrowly defined legal framework; especially if personal profiles are to be created in the process. One crucial aspect is to find an appropriate organisational structure for the data pool. This is not only necessary in terms of defining the responsible entities: it can also allow certain processing activities to be carved out from the strict requirements of applicable data protection law by anonymising data through an appropriate organisational setup, for example, by making use of a data trustee.
Another important step in order to ensure the success of a data pooling project, is to define the use cases from the outset, as specifically as possible. This is because the legal requirements can vary significantly depending on the actual data used, the specific purpose pursued and the entity that will benefit from the analysis. Defining the use cases accurately requires a close co-operation between data scientists, business stakeholders and legal experts.
Data pooling governance and management
A key legal risk relating to data pooling is unrestricted access to a large amount of data: this can result in abuse, such as using the data in ways that breach legal or contractual restrictions. Depending on the nature and source of the data, use of that data may be restricted due to intellectual property rights (for example, licence restrictions), contractual restrictions (including on use, transfers and storage location), privacy requirements (for example, purpose limitation) and data localisation laws restricting the transfer of data outside the territory in which the data was generated. Such use may also give rise to competition issues (for example, information exchange between competitors).
Understanding the limitations on data use at the outset of a data pooling project is critical to determining how best to structure, manage and govern such use in a way that minimises risks to the business but maximises the value of the data. This includes determining where to store the data (central lake vs. local hosting environments), setting clear parameters on purposes for use, establishing access controls, implementing the right security measures and/or anonymisation techniques.
Challenges seen in practice include identifying exactly what restrictions apply to which data, understanding how the data can be used without breaching these restrictions, and subsequently managing this at scale. Difficulties with overcoming these challenges can cause a data pooling project to subsequently fall apart. The most successful projects are those that understand the limitations on data use at an early stage of implementation (and critical to this is a process for mapping data flows and labelling data according to use) and that build a clear and robust data governance structure.