Tipping the Balance: defining a sustainable vision for artificial intelligence
Published on 4th November 2021
Given the huge energy demands of artificial intelligence, how can businesses ensure that it is a credible technology for their decarbonisation initiatives?
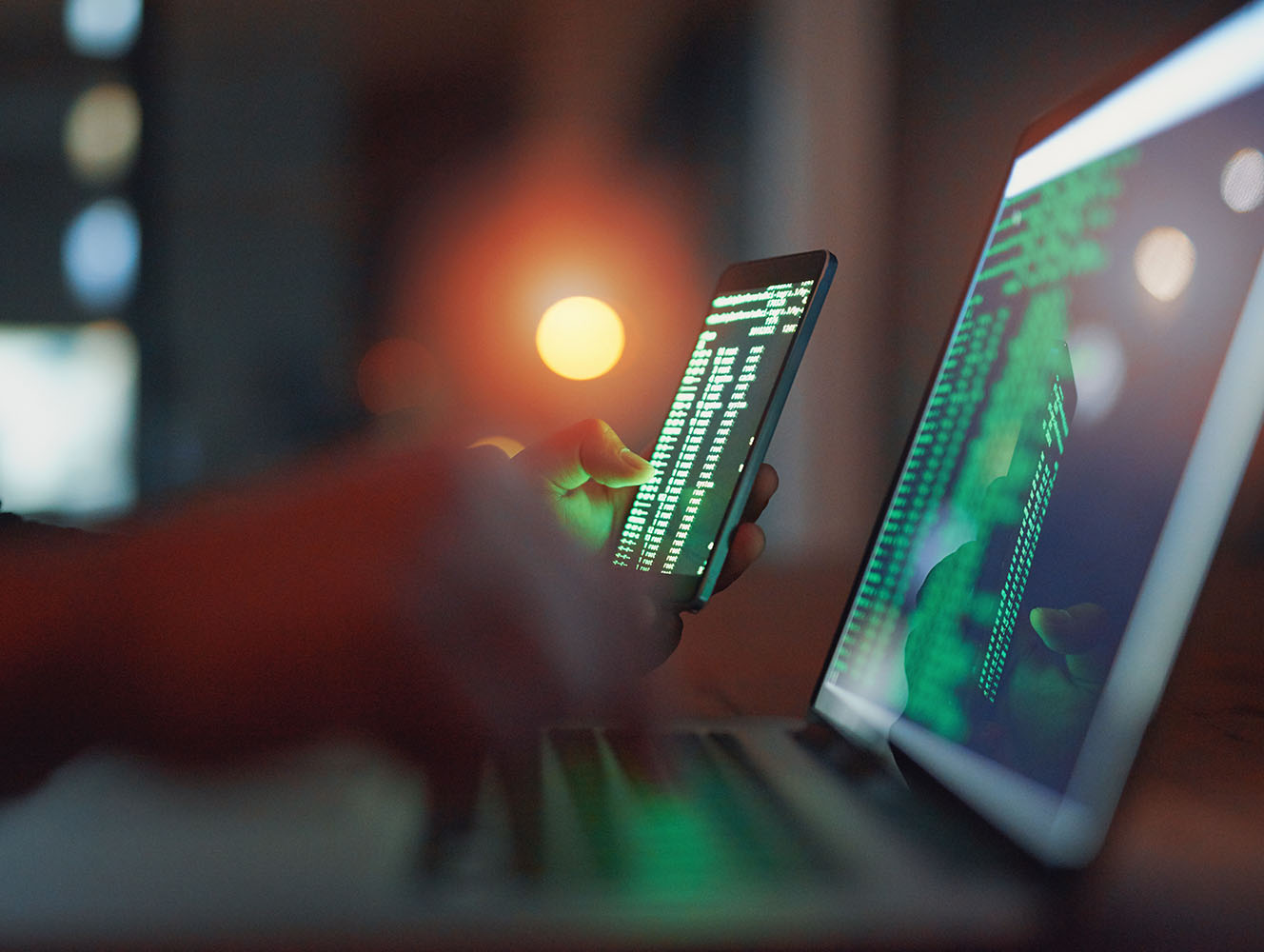
The transformative applications of artificial intelligence (AI) and machine learning are vast and expanding rapidly. Of particular relevance, in the context of the COP26 summit in Glasgow, is its potential to play an important role in supporting decarbonisation efforts across all sectors.
However, machine-learning systems need massive computer-processing power, which in turn needs a huge amount of energy. One commentator calculated that the OpenAI algorithm that learned, through trial and error over a number of months, to manipulate a Rubik's cube may have consumed the equivalent of three hours of electricity output from a nuclear power plant.
Any decision to deploy AI will be a delicate balancing act between the demonstrable benefits of a particular use case as against the embodied carbon generated by its development and operation.
Supporting decarbonisation
AI is not a "silver bullet" in its own right for decarbonisation but, depending on its use, can have a tremendous indirect impact on environmentally protective solutions. Four broad ways have been identified in which AI can support greener and more sustainable products, services and processes.
First, it can distil vast quantities of data into actionable insights. Deforestation or biodiversity can be monitored from automated visual-recognition analysis of satellite images from space. Greenhouse gas emissions can be monitored from satellite or aerial imagery, using advanced cameras that can pick up their observable interactions with sunlight. Patterns can be found in data that might otherwise be too vast to be manageable.
Secondly, it can be used to optimise complex systems, including energy-intensive systems, whether those are domestic heat-generation systems, or complex manufacturing processes.
Production runs can be scheduled in the most efficient way to reduce energy consumption, and opportunities can be identified to re-engineer processes to be more efficient. Waste can be sorted to remove recyclable materials using image recognition, and discarded electronics can be dismantled to retrieve reusable components or materials (waste robotics are one of the 12 decarbonising technologies for cities identified in our "Sustainable Disruption" research with Economist Impact). Energy costs and the systems themselves can be optimised with AI. Transport systems can be modelled better in order to be understood more completely and run more efficiently, and freight routes can be optimised.
Thirdly, AI can be used to improve predictions. Google's DeepMind recently announced that its AI analysis of climate data from the UK's Met Office had produced the most accurate short term weather forecasts for rainfall in the next two hours. Weather forecasts can also support energy-grid balancing by identifying times when renewable generation sources are likely to be oversupplying or undersupplying the grid compared to likely demand, improving grid balancing through storage management. Better demand forecasting can reduce waste from overproduction. Even more ambitiously, the European Commission is financing Destination Earth (DestinE), a high-precision digital model of the Earth. It will focus on the effects of climate change, biodiversity and extreme weather events, and help to predict major environment disasters with increased accuracy.
Finally, AI can accelerate scientific modelling and discoveries. By modelling known data, AI is able to generate predictions for researchers about other ideas that might meet a defined goal, focusing research into areas most likely to prove successful. This can, for example, feed into new greener catalysts for chemical processes that reduce the amount of energy needed by the production process. Advances in battery technology are a particular focus for research and, again, AI can speed up the process by identifying the most promising materials for further testing.
Clearly, AI will not solve climate change in itself – it is only a tool. But there is no lack of variety in the many contributions that AI can make to addressing the decarbonisation challenge.
Funding AI insight
Not surprisingly given its extraordinary potential, there is no lack of funding for AI. Statista estimates that AI funding in the first half of 2021 totalled $38 billion, already greater than total funding across the whole of 2020.
Meanwhile, climate tech and green tech are an unquestionable focus for funders currently. Recently, Forbes forecast that $49 billion will "pour into climate tech in 2021" and Blackrock's chairman and CEO, Larry Fink, predicted that the next 1,000 unicorns (that is, start-ups worth more than $1 billion) will be in climate tech.
Against that background of heavy investment in both AI and climate tech, we would hope that AI-centric climate tech will not struggle to find funding, especially given the push at COP26 for private funding in low-carbon technologies .
The embodied carbon problem
But one issue which could – perhaps should – cause investors to pause is the issue of the massive energy consumption of AI and the risk that its valuable insights come with a huge burden of embodied carbon.
Machine learning relies on big data and operates by building complex multi-dimensional patterns of a particular thing, task, question or process. The pattern is built up and refined by performing extensive mathematical calculations on each individual piece of data from a data set that is passed through the system. The calculations that flow from each piece of data cause the details of the patterning to be recalibrated. This process is repeated as many times as there are instances of data. The more data, the better the model will become, so vast quantities of data are needed for accurate models.
Moreover, the modelling process is speeded up by increasing the processing power applied to it. The low cost and ready availability of vast cloud-based processing capacity means that AI systems can become ever larger, with a correspondingly ever-larger demand for energy.
Can this contradiction between the value of AI's insights and predictions, on the one hand, and its heavy environmental footprint, on the other, be resolved?
Renewable and green energy
A high level of energy consumption does not necessarily translate into a huge carbon footprint.
Businesses are increasingly contracting with energy suppliers to ensure that the power that they consume comes from renewable sources. Many cloud services providers, in particular, have made this a central plank of their decarbonisation strategies. Indeed, the secure streams of future revenues that are generated by these arrangements are a significant factor in financing the growth of renewables infrastructure in some countries.
In addition, data centres are increasingly located in colder parts of the world to reduce the power needed to cool the server racks, with innovations such as underwater data centres being trialled. In the right location, there may be scope to use heat exchange technologies to support cooling (for example in coastal areas where cold seawater can be accessed).
While these strategies are generally works in progress at present, there are notable efforts to move a significant proportion of cloud services to a net zero footing by the end of the decade.
Even where fossil fuel cannot yet be avoided for power generation, technologies around carbon capture, utilisation and storage enable its impact to be reduced. In addition, alternative fuels such as (green) hydrogen are a focus for innovation, research and development as alternatives to fossil fuels.
Although it is not true, today, to say that the majority of AI processing in the cloud is powered by renewable or green energy, this is the clear direction of travel.
Sustainable AI: The need for a clear impact assessment
Pending a comprehensive global switch in energy generation from fossil fuels to renewable sources or alternative fuels such as green hydrogen, businesses seeking to develop or deploy AI tools to support decarbonisation essentially need to make an assessment of whether the environmental benefits of using an AI tool outweigh its carbon footprint.
Whether this assessment can be made in detail will depend on how carefully the AI developers and others in its supply chain have calculated their environmental position. This is an area that is, at present, primarily dependent on corporate policy.
Although in some areas, regulation requires an environmental assessment of products, services or investments, this is not the case across the board. It is notable that, despite the strong EU policies and priorities around tackling climate change, there is nothing in the European Commission's proposed draft AI regulation that addresses the issue of sustainable AI – notwithstanding the extensive and onerous reporting and evaluation requirements that the legislation would impose on AI suppliers in other respects. Given the increased urgency and impetus given to environmentally sensitive and sustainable technology development, it would be surprising if this missed opportunity were not addressed in later drafts of the regulation.
This is clearly an area where customers and investors can apply pressure on developers and suppliers of AI through their purchasing and investment decisions, and through their negotiating red lines in dealing with such businesses.
Given the breadth of ways in which AI can positively support the delivery of decarbonisation initiatives, it would be a tragedy if a lack of sustainable vision in its deployment ultimately undermined those advantages.